Hi!I am a first year Master student at University of Electronic Science and Technology of China. My advisor is Shuhang Gu. I received the B.S. degree from the Artificial Intelligence School, Xidian University in 2023.
My research interest is low-level vision, such as image/video restoration, image enhancement and so on. Specifically, I’m excited about how to build more efficient models, including training and inference, in the field of low-level vision. In addition, I believe that this will ultimately impact the development of the AI community.
If you have any suggestions for cooperation in the low-level vision, please feel free to contact me.
🔥 News
- 2024.03: 🎉🎉 Our work “Video Super-Resolution Transformer with Masked Inter&Intra-Frame Attention”(MIA-VSR) is accepted to CVPR 2024.
- 2024.03: 🎉🎉 Our work “Improved Implicit Neural Representation with Fourier Reparameterized Training”(FR-INR) is accepted to CVPR 2024.
- 2024.03: 🎉🎉 Our work “Transcending the Limit of Local Window: Advanced Super-Resolution Transformer with Adaptive Token Dictionary”(ATD-SR) is accepted to CVPR 2024.
📝 Publications
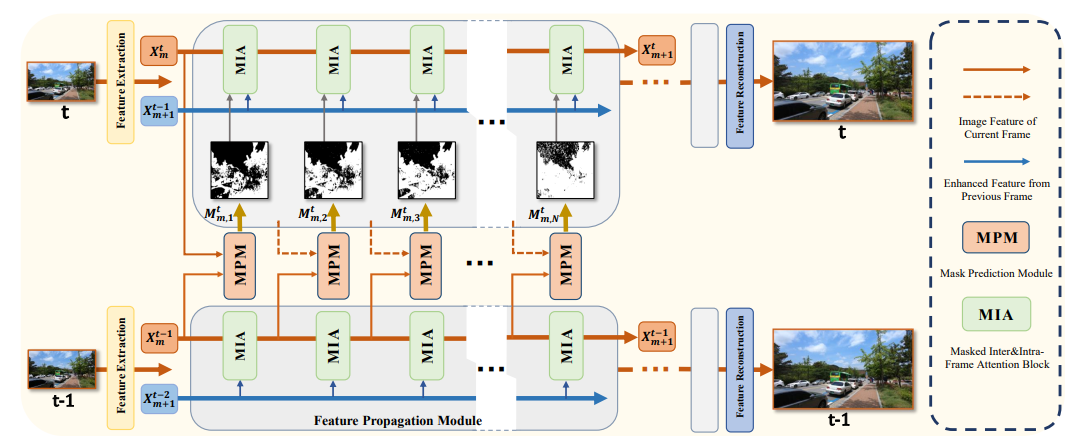
Video Super-Resolution Transformer with Masked Inter&Intra-Frame Attention
Xingyu Zhou, Leheng Zhang, Xiaorui Zhao, Keze Wang, Leida Li, Shuhang Gu
- Focus on the heavy computational burden as well as the large memory footprint hinder the deployment of Transformer-based VSR models. With our proposed masked intra-frame and inter-frame attention block, our MIA-VSR improves the memory and computation efffciency over state-of-the-art methods, without trading off PSNR accuracy.
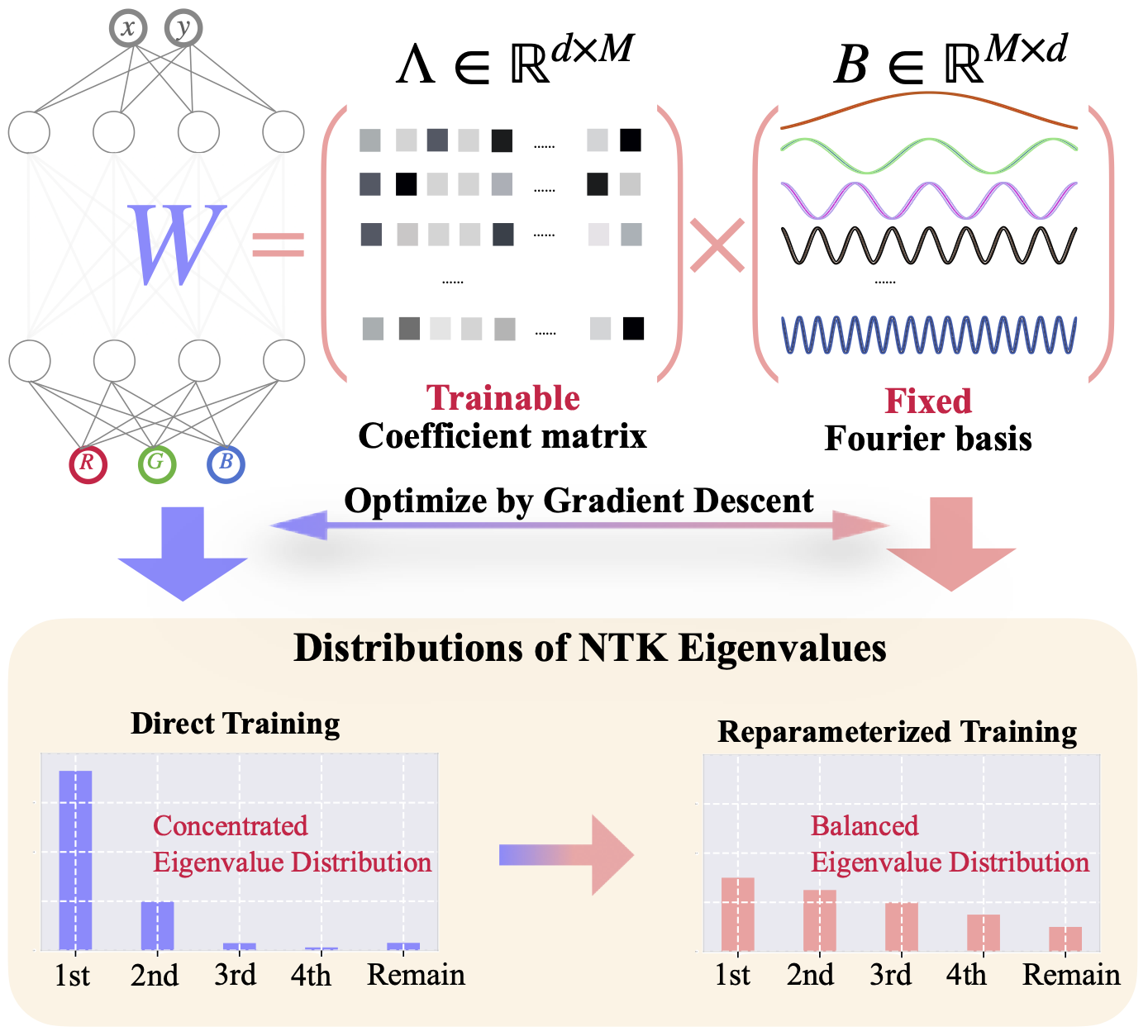
Improved Implicit Neural Representation with Fourier Reparameterized Training
Kexuan Shi, Xingyu Zhou, Shuhang Gu
- Focus on the low-frequency bias issue of vanilla multi-layer perceptron (MLP) in the Implicit Neural Representation (INR). Armed with our Fourier reparameterization method, better INR with more textures and less artifacts can be learned from the training data.
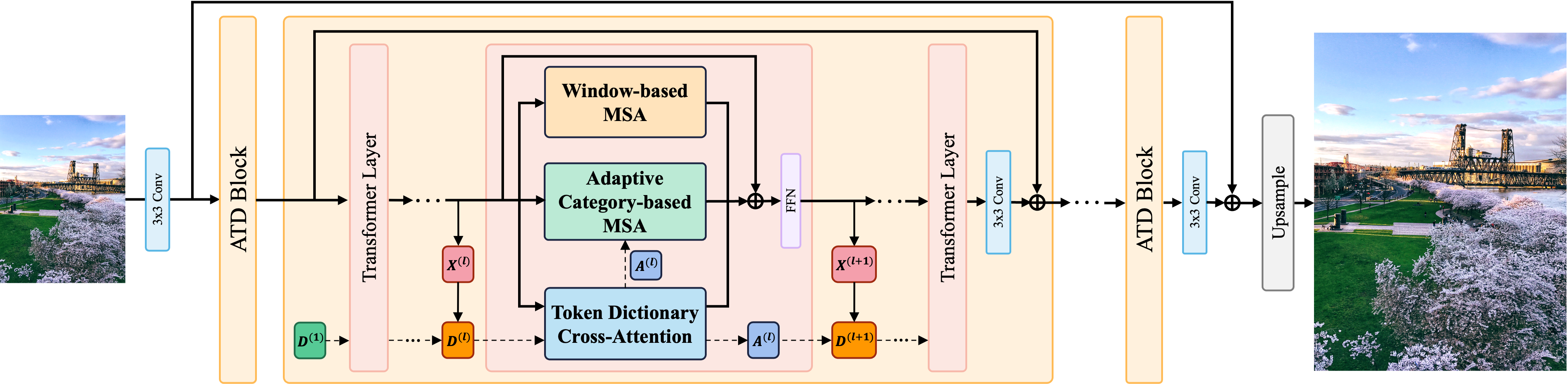
Leheng Zhang, Yawei Li, Xingyu Zhou, Xiaorui Zhao, Shuhang Gu
- Focus on the limited receptive field caused by window-based self-attention in image super-resolution. With introducing a group of auxiliary adaptive token dictionary, our method achieves the best performance on various single image super-resolution benchmarks.